
A reliable disaster recovery approach regularly tests how workloads fail and validates recovery procedures. This is often referred to as horizontal scaling (number of nodes) and vertical scaling (size of nodes).Īn enterprise-wide disaster recovery strategy for most applications and systems requires an assessment of priorities, capabilities, limitations, and costs. Once the peak is over, resources can be freed up and costs reduced accordingly. Adding new resources as needed must be easy, and only actual consumption should be charged for. For an organization to handle all of these workloads, it needs a scalable storage and compute platform. However, new projects, seasonal tasks, or advanced approaches such as model training (for churn, forecasting, and maintenance) create spikes in resource requirements.
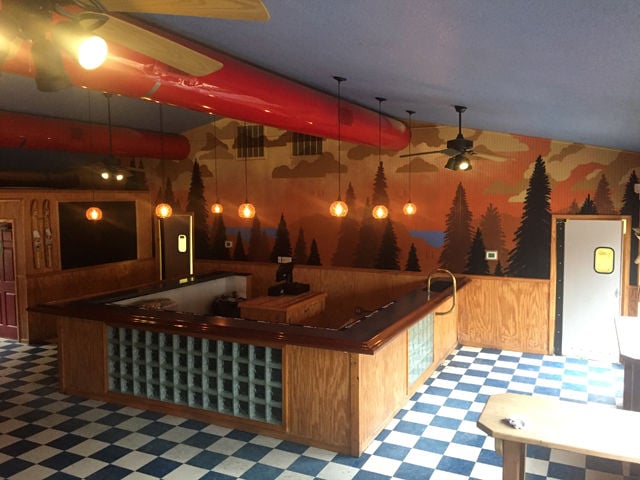
Standard ETL processes, business reports, and dashboards often have predictable resource requirements in terms of memory and compute. It must be actively managed to improve the quality of the final data sets so that the data serves as reliable and trustworthy information for business users. Data quality has many dimensions, including completeness, accuracy, validity, and consistency.
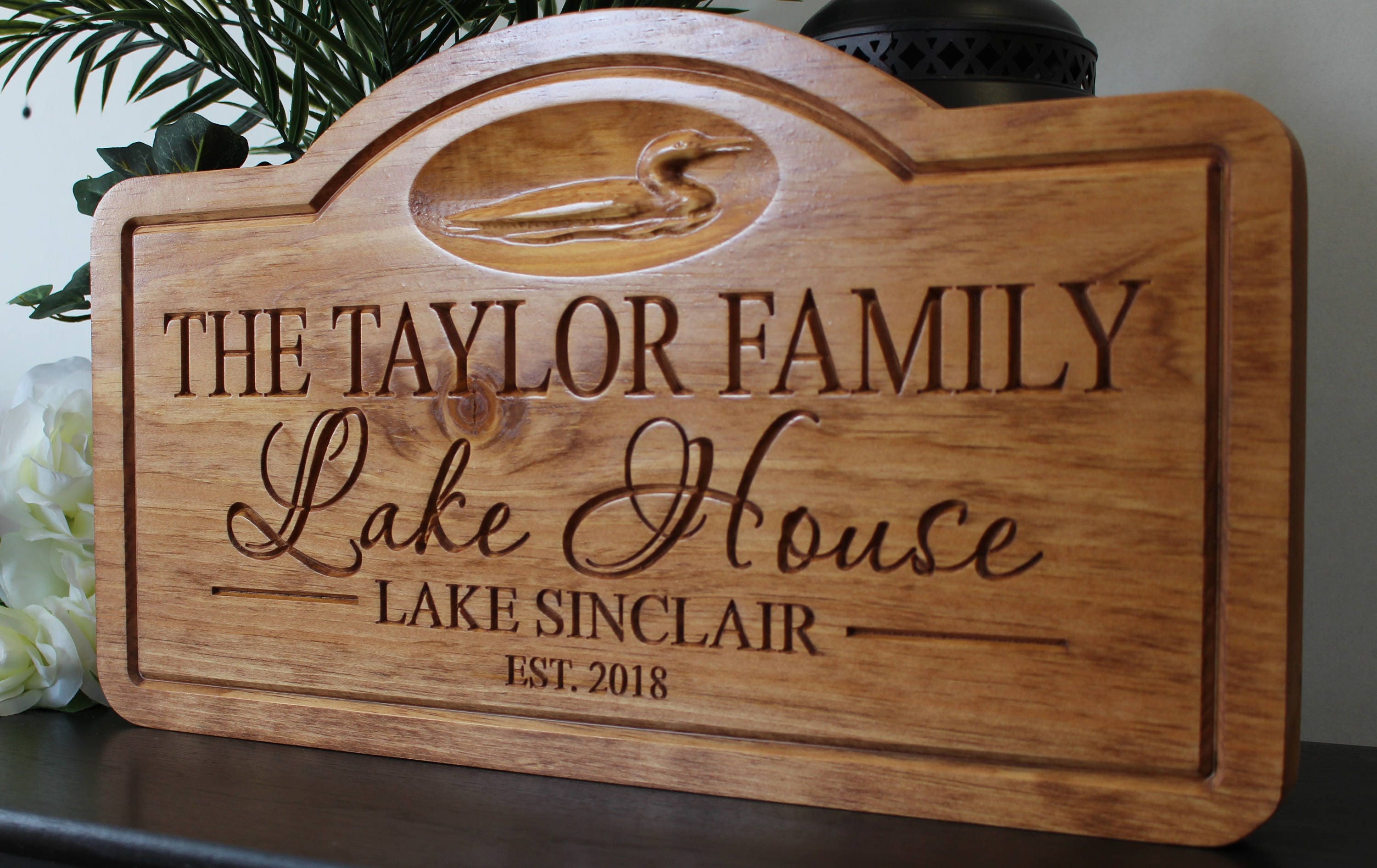
The focus is on designing applications to recover quickly and, in the best case, automatically.ĭata quality is fundamental to deriving accurate and meaningful insights from data. For both the platform and the various workloads - such as streaming jobs, batch jobs, model training, and BI queries - failures must be anticipated and resilient solutions must be developed to increase reliability. In a highly distributed environment, outages can occur.
